Jeremy Combe
Data Scientist
On-Prem text translation from Luxembourgish to English
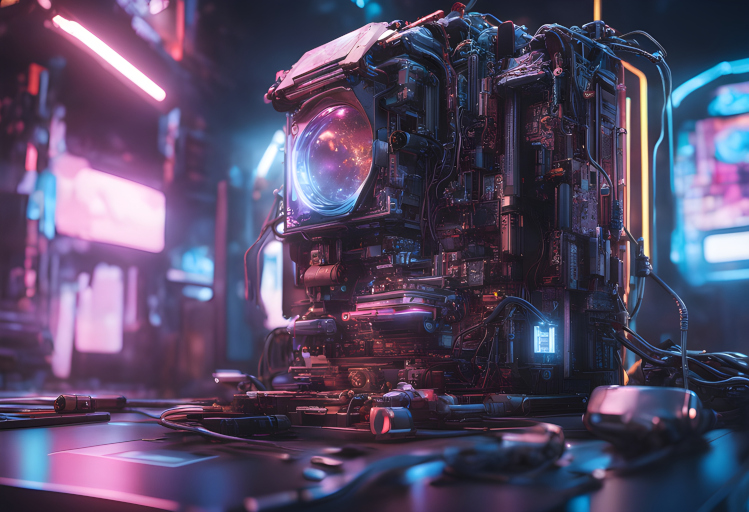
In this post, we will delve into the available solutions for translating Luxembourgish text to English.
Why, you may ask? Well, the options are limited, with only a handful of services designed for this specific task. Furthermore, most solutions either rely on cloud-based services, requiring an internet connection, or come with relatively high licensing costs.
For those seeking alternatives, the evolving landscape of computing devices in recent years has given rise to powerful machine learning models capable of efficiently translating Luxembourgish to English on-premises. What’s more, some of these models are open source.
The goal of this post is to examine, and compare three prominent open-source machine learning models: NLLB-200, OPUS-MT, and MADLAD-400.
But first, we need a way to evaluate different models and compare them properly.
How to evaluate translators ?
Before delving into translation models, it’s crucial to understand why evaluation metrics matter. These metrics serve as objective tools to measure “how good” is the overall quality of machine translation systems. The most commonly used are Chr-F, BLEU, METEOR, and TER.
Here we focus on Chr-F (Character N-gram F-score), a metric emphasizing character-level precision, recall, and F-score which aligns with our goal of capturing linguistic nuances in this specific translation task. It provides a balance between precision and recall, giving equal weight to false positives and false negatives. The F1 score ranges from 0 to 1, with a higher score indicating better performance.
NLLB-200
Meta AI’s revolutionary NLLB-200, introduced in July 2022, stands as a milestone in machine translation with its capability to translate across 200 languages. NLLB-200 extends its support beyond major languages to include 55 African languages, while also covering Luxembourgish. The acronym NLLB stands for ‘No Language Left Behind’, a testament to its commitment to inclusivity.
NLLB-200 isn’t a one-size-fits-all model; instead, it comes in various declinations, each tailored to specific requirements. Examples include NLLB-200-3.3B, NLLB-200-distilled-600M, and NLLB-200-distilled-1.3B, where the final variable indicates the count of parameters.. These variations ensure flexibility in catering to diverse translation needs, offering solutions that align with both computational resources and specific language nuances.
Rigorously evaluated, NLLB-200, in its 3.3B declination, outperforms previous benchmarks by an average of 44%, setting a new standard for language accessibility.
Luxembourgish to English Translation Performances
NLLB-200 demonstrates exceptional performance in translating Luxembourgish to English, showcasing a remarkable accuracy that goes beyond literal translation. Its nuanced understanding of context allows it to adeptly handle idiomatic expressions unique to Luxembourgish. This proficiency sets NLLB-200 apart from many existing tools in the translation landscape.
Notably, the Chr-F for Luxembourgish to English translation with NLLB-200-3.3B stands impressively at 0.71, reflecting its high precision and fluency in capturing the essence of the source language.
Licensing
NLLB-200 operates under the CC-BY-NC license, denoting the Creative Commons NonCommercial license. This license empowers copyright holders to grant public permission for media reuse but strictly for noncommercial activities.
It’s crucial to note that the CC-BY-NC license presents a limitation for users seeking on-premises machine translation solutions. As it stands, the terms of this license may restrict the deployment of NLLB-200 in on-premises environments. This consideration is pivotal for organizations or individuals who prioritize the usage of machine translation models within their local infrastructure.
OPUS-MT
OPUS-MT, developed by the University of Helsinki, stands out with its open-source, community-driven approach. It’s an integral component of the Open Parallel Corpus (OPUS) project, a broader initiative that provides open translated texts for training machine translation systems. OPUS-MT, being part of this larger endeavor, represents a breakthrough in open-source machine translation. Unlike proprietary models, OPUS-MT embraces openness and collaboration, allowing users to continuously improve and customize the system.
Similar to NLLB-200, OPUS-MT is not confined to a one-size-fits-all model. Users have the flexibility to customize their translation models according to specific language pairs and unique requirements. This adaptability is reflected in the extensive community-driven contributions evident in the multitude of OPUS-MT models available. For instance, a quick exploration on HuggingFace unveils a vast collection, boasting over 1500 distinct OPUS-MT models. This remarkable diversity is a testament to the collaborative efforts within the community, showcasing the system’s widespread adoption and interest.
In the context of translating text from Luxembourgish to English, various OPUS-MT models cater to specific linguistic nuances. Examples include opus-mt-mul-en, opus-mt-ine-en, opus-mt-gem-en, and opus-mt-ine-en. Each of these models possesses the capability to translate text from various languages to English. Specifically, opus-mt-gem-en is designed to excel in Germanic languages, while opus-mt-mul-en offers a broader, general proficiency in translating text from various languages to English.
Luxembourgish to English Translation Performances
Among the different versions of OPUS-MT models capable of translating from Luxembourgish to English, opus-mt-ine-en emerges as the optimal choice, especially well-suited for general and less complex texts. Remarkably, opus-mt-ine-en attains a Chr-F score of 0.54 in Luxembourgish to English translation, highlighting its commendable performance.
In contrast, the Chr-F scores for other mentioned versions were lower, underscoring the distinctive strength of opus-mt-ine-en in achieving heightened accuracy and fluency in translating Luxembourgish to English.
Licensing
OPUS-MT operates under a business-friendly open-source license, namely the Apache License 2.0. This license permits the incorporation of the software into any commercially licensed software or enterprise application for free. Users have the freedom to leverage OPUS-MT within proprietary systems without incurring licensing fees.
It’s crucial to note, however, that the usage of Apache trademarks is restricted in licensed proprietary software or any associated legal and organizational documentation. This careful consideration ensures compliance with the terms of the Apache License 2.0 while fostering an environment where businesses can seamlessly integrate OPUS-MT into their commercial applications.
MADLAD-400
Introduced by Google Research in September 2023, MADLAD-400, an acronym for Multilingual Audited Dataset: Low-resource And Document-level, stands as a formidable addition to the machine translation landscape. This model has been designed with flexibility in mind, supporting translations across an impressive array of 419 languages, including Luxembourgish. This expansive language coverage, coupled with translation performances comparable to the groundbreaking NLLB-200, positions MADLAD-400 as a versatile and high-performing solution.
Similar to NLLB-200’s various declinations, MADLAD-400 is available in multiple versions tailored to different requirements. Examples include MADLAD400-3b-mt, MADLAD400-7b-mt, and MADLAD400-10b-mt, each with its specific parameters denoting the number of parameters used in the model.
Luxembourgish to English Translation Performances
In the context of Luxembourgish to English translation, MADLAD-400 lags behind the other two models, recording a Chr-F score of 0.51. See Link for detailed results. While still demonstrating a capability for translation, this score suggests that MADLAD-400 may not match the precision and fluency achieved by the NLLB-200 and OPUS-MT-INE-EN models in this particular language pair. Users may want to consider these differences in performance when choosing a model for Luxembourgish-English translation tasks.
Licensing and Accessibility
MADLAD-400’s license is Apache 2.0, same as OPUS-MT models.
Conclusion
In summary, our examination of Luxembourgish to English translation models highlights the strengths and considerations for three open-source options: NLLB-200, OPUS-MT, and MADLAD-400.
NLLB-200 by Meta AI offers exceptional versatility, translating across 200 languages, with a notable Chr-F score of 0.71 for Luxembourgish to English. However, the CC-BY-NC license limits on-premises use.
OPUS-MT, from the University of Helsinki, emphasizes community-driven openness. opus-mt-ine-en stands out with a Chr-F score of 0.54. This model supports 59 languages, and is under the Apache License 2.0 which permits free commercial use.
MADLAD-400, introduced by Google Research, supports translation for 419 languages. In Luxembourgish to English, it achieves a Chr-F score of 0.51. Its Apache License 2.0 aligns with business-friendly open-source principles.
Note that the metrics provided for NLLB-200 and MADLAD-400 have been obtained against the same dataset, whereas the OPUS-MT results have been obtained against another, potentially causing variations in OPUS-MT results. Users should consider this when selecting models for Luxembourgish-English translation tasks.
Additionally, when prioritizing on-premise usage, it’s essential to account for licensing restrictions, particularly for NLLB-200.